Inhalation of asbestos fibres can cause serious lung damage including asbestosis and lung cancer. When demolishing and renovating old buildings, care must be taken to correctly handle and dispose of materials containing asbestos. To follow the right precautions, it is necessary to be aware of the presence of asbestos both in the buildings in question and in the waste. A non-contact, fast, and reliable detection system is thus of primary importance. In this case study, we demonstrate how hyperspectral imaging can be used to detect asbestos and separate it from other materials in mixed building waste. This technique offers a way of detecting asbestos on-site during demolition and a way of sorting asbestos and other materials mixed with it in-line at the recycling plant for correct handling and disposal. An automated identification and sorting system can greatly reduce the risk of human exposure.
Sample preparation
Five different sets containing five different classes of typical demolition and renovation (D&R) materials were created for this study. The samples were previously identified and grouped to facilitate analysis and modeling.
- Asbestos
- Unknown D&R mix
- Concrete
- Ceramics
- Terracotta
In this study, we have looked at five groups of materials including asbestos from pipes and roof coverings, an unknown mix of rubbles, concrete, ceramic from plates, and terracotta from pots and tiles. The unknown samples are assumed to be made of materials different from those in the known classes. While some of the samples can be identified visually, manual sorting can be a tedious and hazardous job. Automation using RGB-based machine vision is also limited as some critical materials such as ceramics and asbestos cannot be consistently separated based on color imaging only. Instead, spectroscopic information from the samples can be used to distinguish and accurately identify the different materials they are made of.
Spectra acquisition was carried out with a
HySpex Baldur S-384 N camera (930 – 2500 nm) with a spectral resolution of 5.45 nm. Using a
close-up lens with a 1 m working distance and a 40° field of view covering 70 cm on the scene, a spatial resolution of 1.8 mm was achieved. The low keystone and smile aberrations** on the HySpex cameras allow to accurately image pixel-size objects and preserve the spectral fidelity of the materials.
The sample sets were scanned using an industrial conveyor belt directly underneath the camera with broadband halogen lamps used as illumination sources. The system is fully compatible with third-party sorting solutions such as pneumatic or mechanic sorting machines and robots. The system can be used for online real-time classification and separation as well as for on-site inspection from a tripod.
The acquired hyperspectral image data were analyzed using the
Breeze software from Prediktera. A PLS-DA classification model was built with 4 different classes without using the unknown rubbles mix as an independent class. Sample set 1 was used to train the model while sample set 2-5 were used to test its performance.
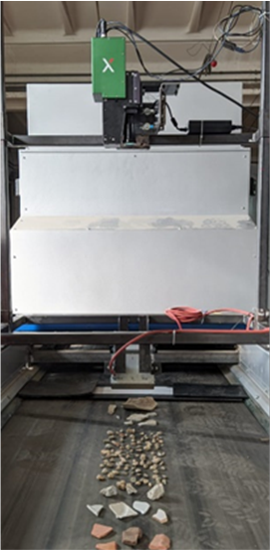
Although the SWIR reflectance spectra of the five different classes look similar, the different materials all have spectral features that are unique enough to separate them from each other and to create a robust classification model. All pieces of asbestos, concrete, ceramic, and terracotta were correctly assigned to their classes – even the smallest ones. The rubble pieces were of varying type and hence not given their own class. The majority were correctly detected as no class but some were identified as part of one of the other classes. This could be either false positives or misidentification during the preparation of the samples. If needed, the varying spectral properties of this group can be analyzed in detail and included in the model.
- Asbestos
- Unknown D&R mix
- Concrete
- Ceramics
- Terracotta
Results from the PLS-DA classification The different materials were accurately classified by the model created in
Breeze. The low keystone of the camera allows identifying objects just a few pixels large while the low smile of the system permits to build robust models using the small spectral differences between the classes.
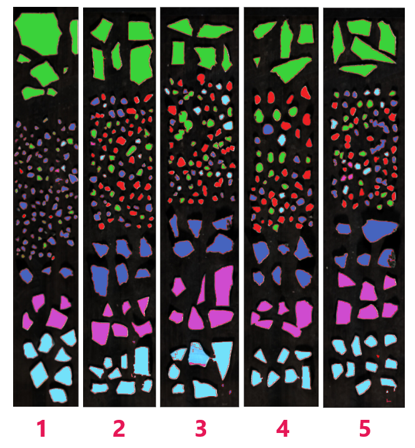
This example shows that hyperspectral imaging can be used to safely detect asbestos and that asbestos can be distinguished from other typical D&R materials. This can form the basis for an automatic sorting system where humans don’t need to handle the potentially hazardous waste. Concrete, ceramic and terracotta can also be separated if further sorting is required. The example also shows that this application benefits from the high spatial and spectral resolution and low optical aberrations achievable with HySpex cameras.